Roles of AI in Science and Engineering—Part 1
- Pisek Kultavewuti
- Jul 1, 2023
- 5 min read
As a photonics engineer by education, expertise, and passion, I find joy in toying with the laws of physics and have built hardware like smartglasses and laser engines. In 2017, I expanded my passion into the realm of AI and machine learning (ML), and have greatly benefited from both learning about AI and applying it to my engineering work. In this two-part series, I wish to share how you, especially if you're a hardware engineer, can benefit from AI. For this article, the Part 1, we will go into comparing the concepts of AI and science and highlight AI's strengths with examples of how AI is progressing science.
Quick Primer on AI
Let's start by discussing the similarities and differences between science/engineering and the field of AI. This is essential to understand how they can complement each other in advancing human technologies.
A practical definition of AI could be as follows: AI is a program created to complete a specific task, such as detecting objects in an image, summarizing text, or navigating a robot through a maze. The AI model is a structure of logic or math containing a set of values needed for certain computations. The key point is that these necessary values are established during the 'training' of the AI model, implying that our AI model is created from the dataset we have. AI models aim to 'model' the dataset provided during training.
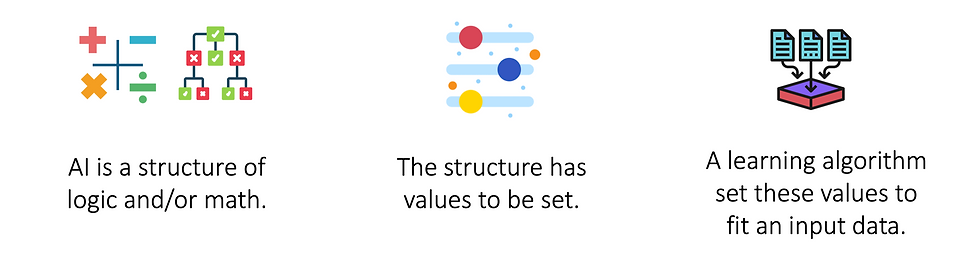
The Parallels Between Science and AI
In terms of modeling, AI experts create AI models from datasets, while scientists (and engineers, by extension) often create or revise theories and laws in their fields. They ‘model’ how the universe works from their dataset of observations.
For example, Sir Isaac Newton proposed the law of gravity (F=GMm/r^2) by observing and pondering why an apple fell. Similarly, Charles Darwin modeled his observations of different species in different places, forming the theory of natural selection.
In essence, scientific theories are analogous to AI models, and scientific observations are similar to AI's input datasets.
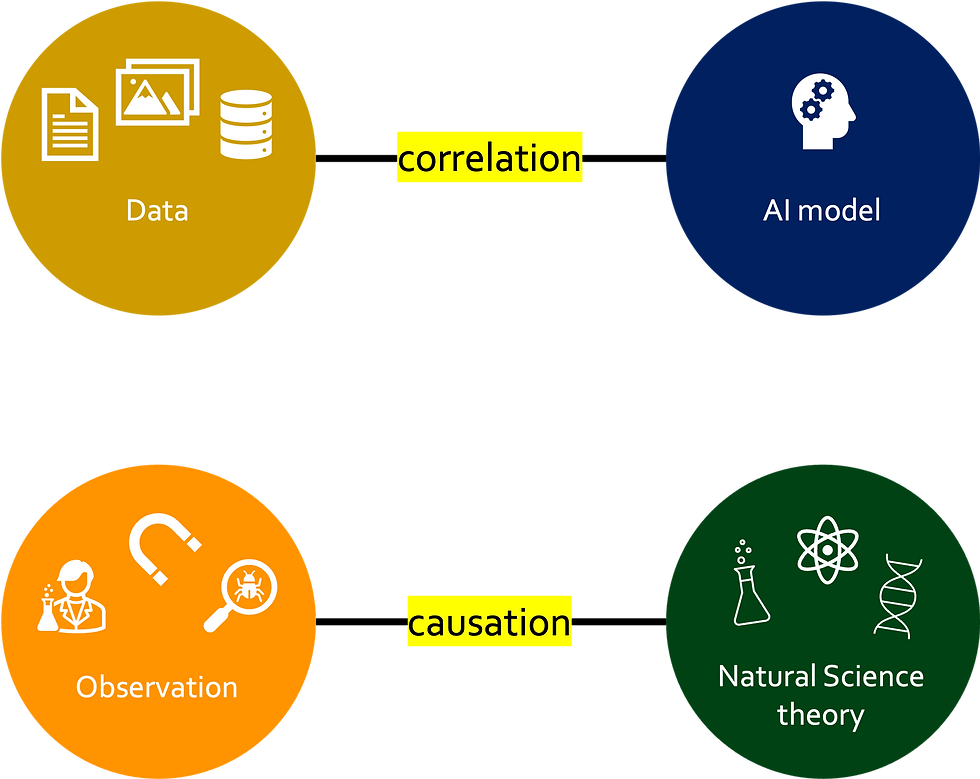
Correlation vs Causation
However, there is a key difference worth realizing. In general, when an AI model is created from a given dataset, the AI model learns to ‘correlate’ inputs to outputs; they do not reason for cause and effect.
For example, an AI model trained to detect humans or animals in an image can correlate a certain distribution of colored pixels to the presence of a human or an animal. This AI model can incorrectly detect a giraffe if a human dresses up in a certain way. Another example is banks using an AI model to determine if a loan applicant is likely to default on a new loan. Banks create such models from a client’s data, including gender, age, number of children, education, occupation, salary, and previous loans. At best, the model can only correlate the risk of default to this information. There is no guarantee, for example, that having a high salary reduces the chance of defaulting on a loan.
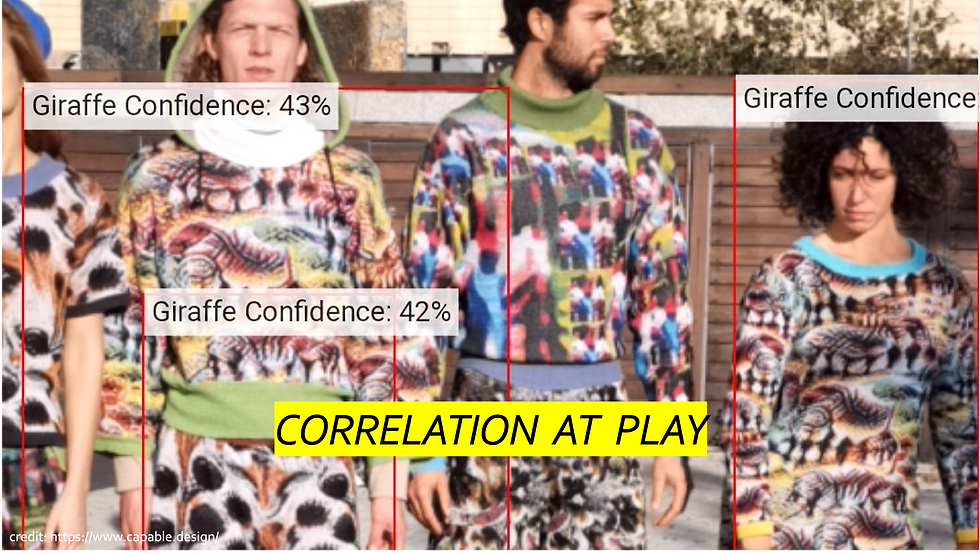
In stark contrast, when a scientist creates a theory, they seek to expose ‘causations’ between inputs and outputs: when inputs are satisfied, outputs are inevitable like a domino effect. Bringing north and south magnets close together, they attract each other. Igniting air containing hydrogen and oxygen will result in fire. When light travels sufficiently near a black hole, its trajectory is bent. Not all theories are perfectly correct. However, scientists commit to unearth causation rather than settling with superficial correlation.
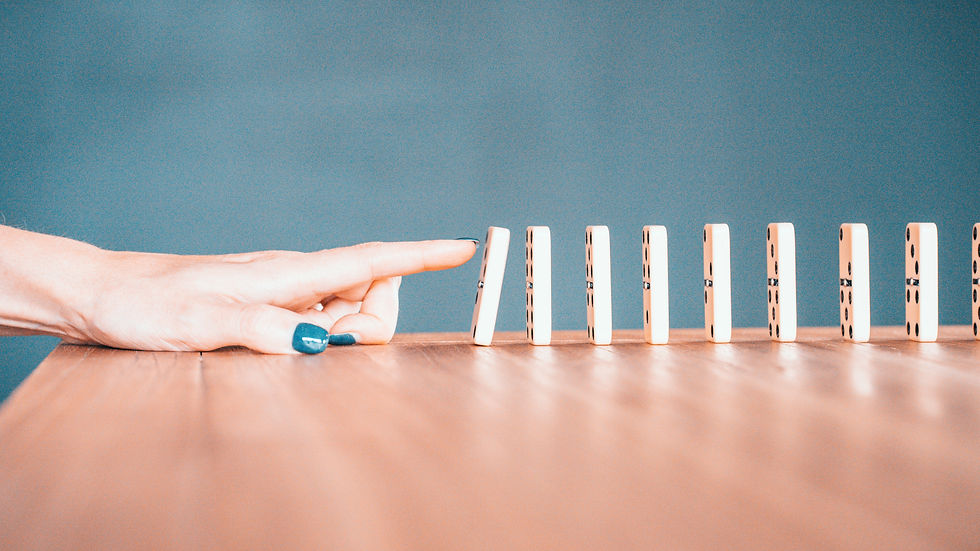
The Power of Causation = Simulations
Why does causation matter? It allows us to simulate situations quite accurately, especially in situations that are rare, expensive, or dangerous. Electrical theories allow us to simulate how electrical devices work rather than building them and seeing what happens. We definitely do not want to build a rocket and launch it into outer space without meticulous design and simulation. It would be extremely expensive and dangerous if rockets explode and crash into cities. Molecular simulations greatly aid drug discovery and manufacturability since actual production takes a lot of time and resources. Thus, the natural laws that scientists and engineers hone their expertise on allow them to simulate and optimize their designs and discoveries.
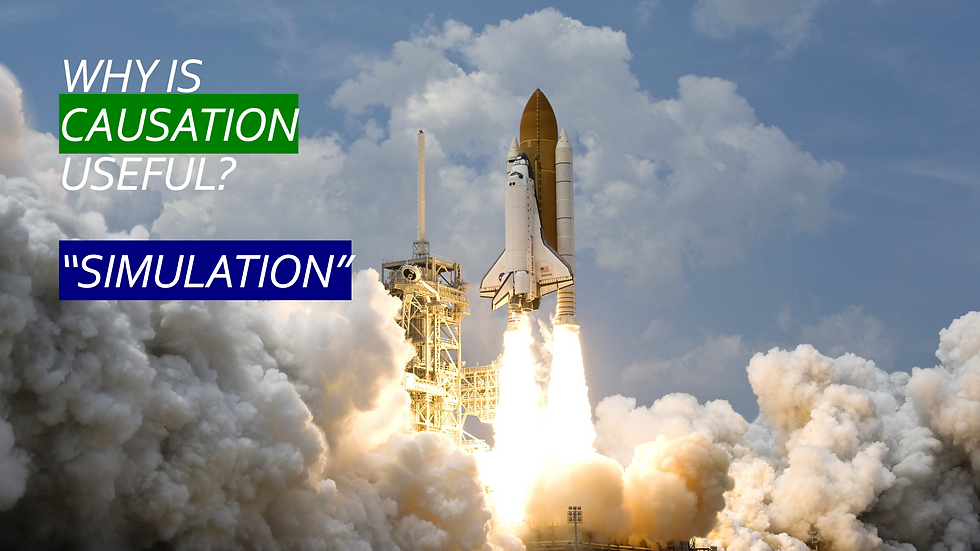
Complexity of Simulation Software
Simulation software exists, but most come with a lot of challenges. Scientific theories are usually universal and can be adapted to any imaginable situation. For example, the law of forces and motion can describe what happens on a pool table: balls colliding with one another and the rails. In simulation software, however, users need to describe the situation in detail. This could include information about the balls (size, mass, surface friction), information about the rails (surface friction, elasticity), and information about the bed cloth or felt (friction, flatness).
The amount of information required in the simulation increases with the complexity of the situation and the level of detail the user wants in the calculation. Thus, using simulation
software often feels like a regular passenger entering a cockpit to fly a plane.

Engineers often encounter the following challenges when using simulation software:
Setting up a scene is rather manual, requiring engineers to select interactive entities and provide correct parameters. Manual processes increase the risk of human error.
If a scene simulation involves many natural laws (i.e., total physics simulation), engineers could face a large number of parameters and couplings between these laws. As many laws are studied in isolation, such as gravity and thermodynamics, one simulation could require experts from various fields.
Simulation software usually treats different laws or cases with different toolboxes or plugins. These toolboxes have several critical parameters, often represented with a symbol. Engineers can be confused if several toolboxes use similar symbols, and junior engineers can use the wrong toolbox altogether.
The amount of calculation result can be very large if the user wants the calculation in detail. Engineers face a challenge in analyzing such data in a timely manner.
Impact and Progress of AI in Science and Engineering
Scientific and engineering challenges occur daily. They could be at a cosmic scale, like searching for habitable planets, or at a quantum scale, like tracing subatomic particles. Some are closer to our lives, such as creating new effective drugs for a certain illness.
AI's Key Strengths
AI can be a powerful tool in handling these challenges. Its strengths can be grouped into the following categories:
Big data. AI can learn patterns, summarize information, or extract details from a huge dataset.
Automation. AI could handle a series of complex tasks with minimal requirement of human oversight.
Exploration. AI is tireless, and with proper setup, it can explore regions that the human mind might not be able to handle.
Speed. Well-calibrated AI, coupled with good hardware, can finish a given task much faster than a human can.
Scale. AI starts to outperform manual effort when the task involves a large number of entities, be they customers, blood samples, or galactic stars.
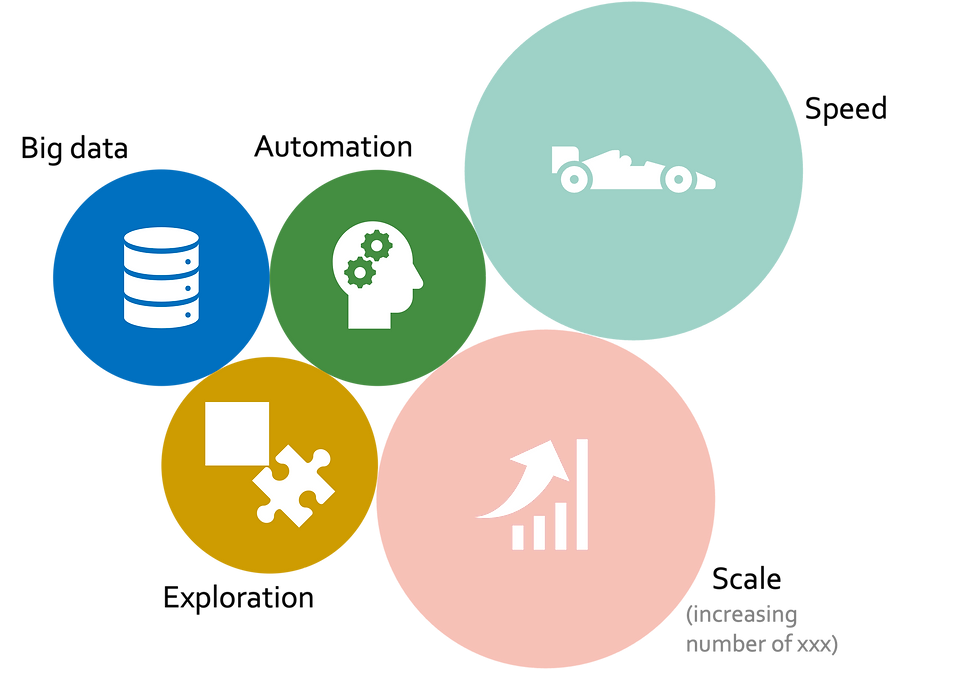
Examples of AI's significant impacts and advancements
The use of humanoid robots, like those developed by Boston Dynamics, which can assist on construction sites or perform hazardous tasks.
AI systems designed to analyze vast amounts of data from outer space; given the Milky Way alone contains about 1 billion stars, this is no small feat!
AI's critical role at CERN where it aids scientists in analyzing and tracking sub-atomic particles generated from high-energy particle collisions, handling data volumes that reach terabytes per day.
In genomics, AI assists scientists by deciphering patterns within voluminous genomic data, a task that is becoming increasingly demanding as DNA sequencing and related tools continue to advance.
The field of chemical and molecular design has also benefited from AI, where it is used to visualize, predict properties, and identify optimal molecular structures.
Thank you for reading this far. I hope you have a good grasp on the interplay between AI and science, and you, perhaps as an engineer, start to see how AI could take your career to the next level.
In the next article, I will give specific examples of what tasks and how AI would make impact in the engineering work by presenting it with a real-world case.
Comments