AI, ML, DL-Practical Definitions
- Pisek Kultavewuti
- Jun 9, 2023
- 2 min read
Updated: Jul 3, 2023
Welcome to the realm of artificial intelligence (AI), where a cascade of acronyms and buzzwords compete for attention. AI, ML, DL — they're more than just alphabet soup. They represent fascinating fields of study, are often used interchangeably, but they are not identical. Today, we're taking a deep dive into their definitions and differences.
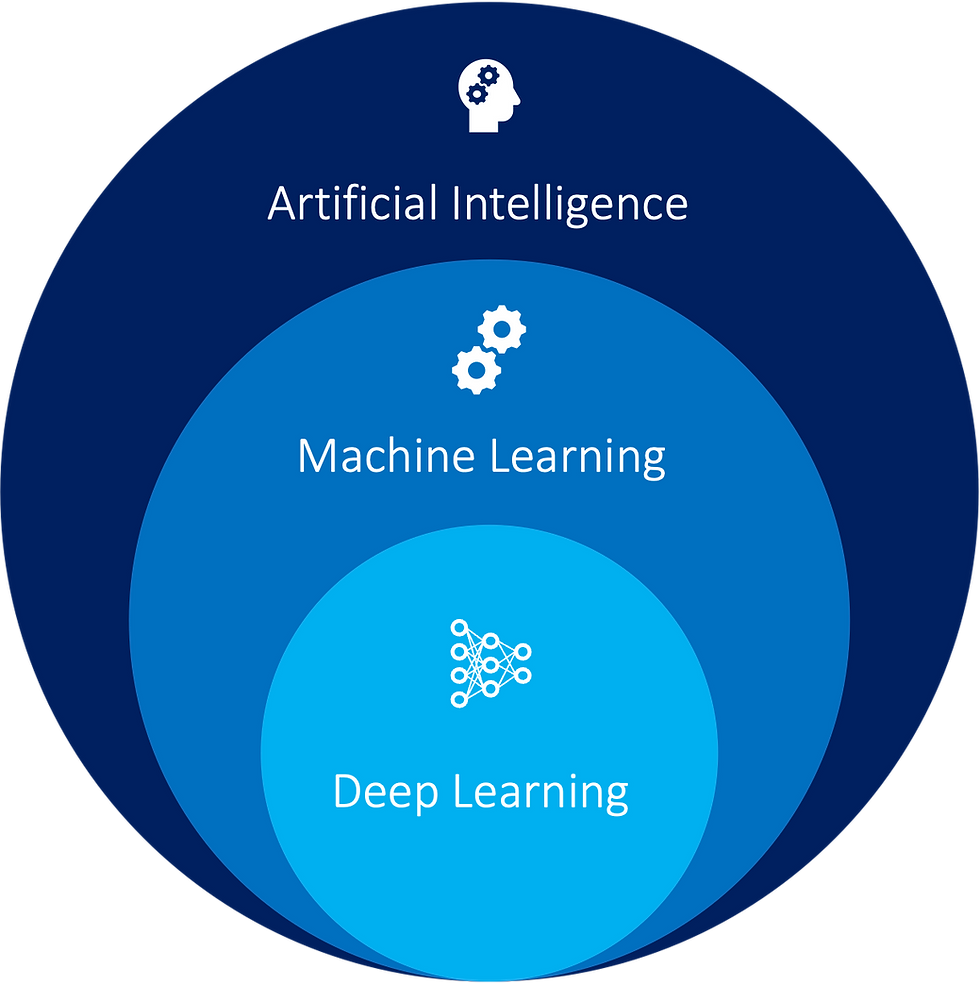
For those in a hurry, here goes the summary of summaries:
AI, or artificial intelligence, is the encompassing theory that guides the grand quest of making machines capable of human-like thought. Consider it the mother ship, the umbrella, the vast overarching category under which all others reside. ML, or machine learning, is one of many approaches conceived to achieve AI. Inside the ML field, DL or deep learning is a kind of machine learning whose structure is inspired by how human brain is wired and works.
If you're staying for a deeper dive, let's go.
Artificial Intelligence
AI comes in varying degrees of sophistication.
At one end, we have 'weak AI,' or ANI (Artificial Narrow Intelligence). These AIs are specialists, honed to perform specific tasks, such as image recognition or autonomous driving. They're the quiet workhorses of our digital age, running the gamut from Siri on your iPhone to the recommendation algorithms on your favorite streaming service. Even ChatGPT falls into this classification.
Progressing up the ladder, there's 'strong AI,' or AGI (Artificial General Intelligence). These AI platforms are designed to mimic human intelligence, possessing the ability to learn, reason, and apply knowledge across diverse domains. While we're still a considerable distance from AGI, a lot of research effort is progressing the field. I would think of a strong AI as the fictional Ironman's Jarvis.
Finally, we have 'super AI,' or ASI (Artificial Super Intelligence). This level of AI, which theoretically would surpass human intelligence in all respects, remains purely in the realm of speculation and imagination, the stuff of Asimov and other science fiction masterminds. Terminator quickly comes to my mind.
Machine Learning
Machine learning is one method, among many, to achieve AI. Metaphorically, if achieving AI is a destination, ML could be a racing car we drive to reach it. ML uses statistics and optimization to turn the dreams of AI into a reality, enabling machines to learn from data, rather than being explicitly programmed.
This field is a wide canvas, accommodating various models like decision trees, support vector machines, neural networks, and k-mean clustering. These models learn from data, tweaking and adjusting their parameters to optimize performance. It's akin to how a student learns from past exams in order to pass the new exam.

Deep Learning
Finally, deep learning (DL) is the recent proverbial monument in the ML landscape. Rooted in the concept of neural networks, DL systems utilize multiple layers of computational nodes, mirroring the way neurons are connected in the human brain. This layered architecture allows DL models to handle complex tasks more effectively.
DL models have delivered stunning breakthroughs, especially in areas like computer vision and natural language processing. Whether it's your phone's camera recognizing your face or a chatbot like ChatGPT interacting seamlessly with you, DL is powering these state-of-the-art applications.
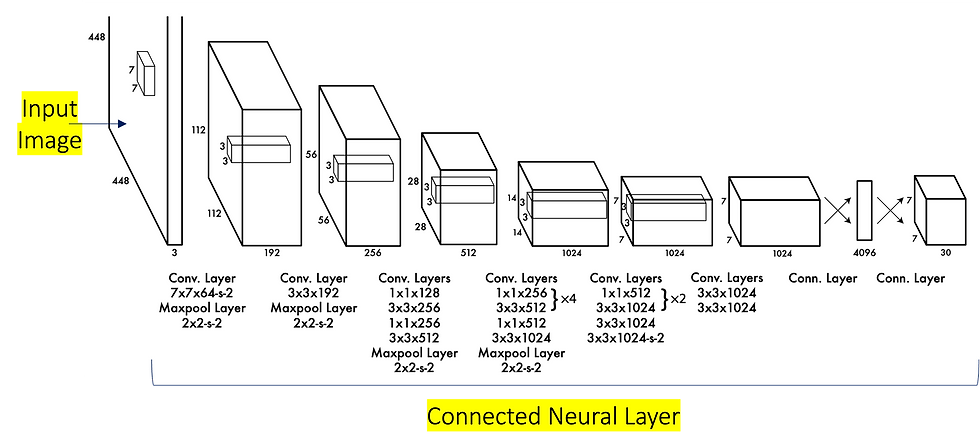
In summary, AI, ML, and DL are in fact interconnected sub-disciplines. AI is the grand vision, ML is the framework that helps achieve that vision, and DL is one powerful tool making significant strides in complex tasks.
亚博体育 亚博体育 亚博体育 亚博体育 开云体育 开云体育 开云体育 开云体育 乐鱼体育 爱游戏体育 华体会体育 华体会体育